AI & Fundamentals
Embodied Learning as a Path to Intelligent Machines - Sergey Levine, Assistant Professor, UC Berkeley
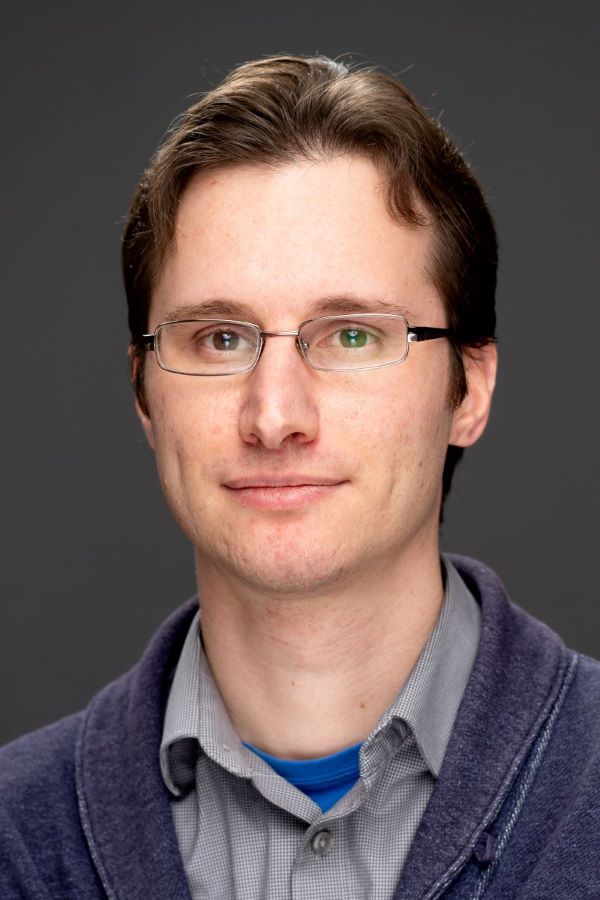
DATE: Mon, December 9, 2019 - 11:00 am
LOCATION: ICCS\CS - X836, ICICS Computer Science, 2366 Main Mall, Vancouver, BC
DETAILS
Abstract:
We've seen machine learning methods succeed in domains where hand-designed methods have proven insufficient: visual perception in open-world environments, speech and text recognition, machine translation, and a range of other real-world passive perception tasks. Most of these success stories have been predicated on the availability of large, hand-labeled datasets. However, the flaws in these existing systems are readily apparent when they are utilized outside of the confines of their test set: while machine learning is remarkably effective at generalizing the patterns in the data, even the largest and most powerful current models are prone to make mistakes that for us seem to defy common sense. I will discuss how the lack of situated or embodied learning is in large part responsible for these failures, and argue that studying machine learning in the context of embodied systems that inhabit the same physical world as us is the key to endowing learning-enabled machines with the kind of common sense that so far has been lacking. Embodied learning typically means learning behaviors and skills, rather than patterns, which necessitates a shift in the algorithmic frameworks that we employ. Reinforcement learning and optimal control offer the algorithmic tools that allow us to get started on this problem, but have a number of major shortcomings that make them difficult to apply to enable the sort of embodied and situated learning that we need to endow machines with common sense. I will discuss some of these shortcomings, and the initial steps that we have taken to begin to address them. The talk will cover our recent research on robotic learning, off-policy reinforcement learning, and meta-learning algorithms.
Bio:
Sergey Levine received a BS and MS in Computer Science from Stanford University in 2009, and a Ph.D. in Computer Science from Stanford University in 2014. He joined the faculty of the Department of Electrical Engineering and Computer Sciences at UC Berkeley in fall 2016. His work focuses on machine learning for decision making and control, with an emphasis on deep learning and reinforcement learning algorithms. Applications of his work include autonomous robots and vehicles, as well as computer vision and graphics. His research includes developing algorithms for end-to-end training of deep neural network policies that combine perception and control, scalable algorithms for inverse reinforcement learning, deep reinforcement learning algorithms, and more.
Host:
Professor Bhushan Gopaluni, Department of Chemical and Biological Engineering, UBC
CAIDA Contact:
Arynn Keane
arynnk@mail.ubc.ca