AI & Fundamentals
Contrastive Learning for ML-guided MIP search - Bistra Dilkina, Associate Professor, University of Southern California
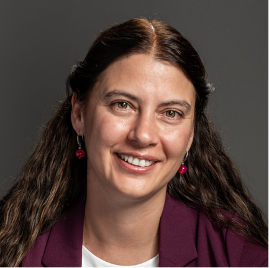
DATE: Mon, December 16, 2024 - 2:30 pm
LOCATION: UBC Vancouver Campus, ICCS X836
DETAILS
Abstract:
Recent research has demonstrated the ability to significantly improve MIP solving by integrating ML-guided components and learning policies tailored to specific problem distributions. This benefits directly the real-world deployment of Mixed Integer Programming models as they are often used to solve repeatedly similar problems, arising within a specific application context. We show that one important aspect of designing an effective ML integration in MIP solving is the training loss utilized to tune the ML model parameters. In particular, many important (heuristic) tasks in MIP solving involve choosing subsets of variables, and we demonstrate that Contrastive Loss is particularly well-suited for this setting by learning from both positive and negative examples of candidate sets. We show the successful application of contrastive loss training in the context of Large Neighborhood Search for MIP, as well as Backdoor selection for MIP, resulting in significant speed-ups over multiple domains. Finally, we introduce d-MIPLIB (Distributional MIPLIB) as a resource to the research community in ML-guided MIP solving in order to facilitate benchmarking across a variety of problem domains and drive progress in this field further.
Bio:
Dr. Bistra Dilkina is an associate professor of computer science at the University of Southern California, co-director of the USC Center of AI in Society, and the inaugural Dr. Allen and Charlotte Ginsburg Early Career Chair at the USC Viterbi School of Engineering. Her research and teaching are centered around the integration of machine learning and discrete optimization, with a strong focus on AI applications in computational sustainability and social good. She received her Ph.D. from Cornell University in 2012 and was a post-doctoral associate at the Institute for Computational Sustainability. Her research has contributed significant advances to machine-learning-guided combinatorial solving including mathematical programming and planning, as well as decision-focused learning where combinatorial reasoning is integrated in machine learning pipelines. Her applied research in Computational Sustainability spans using AI for wildlife conservation planning, using AI to understand the impacts of climate change in terms of energy, water, habitat and human migration, and using AI to optimize fortification of lifeline infrastructures for disaster resilience. Her work has been supported by the National Science Foundation, National Institute of Health, DHS Center of Excellence Critical Infrastructure Resilience Institute, Paul G. Allen Family Foundation, Microsoft, and Qualcomm, among others. She has over 90 publications and has co-organized or served as a chair to numerous workshops, tutorials, and special tracks at major conferences.