AI & Fundamentals
Halting Time is Predictable for Large Models: A Universality Property and Average-case Analysis - Courtney Paquette, Research Scientist, Google Research
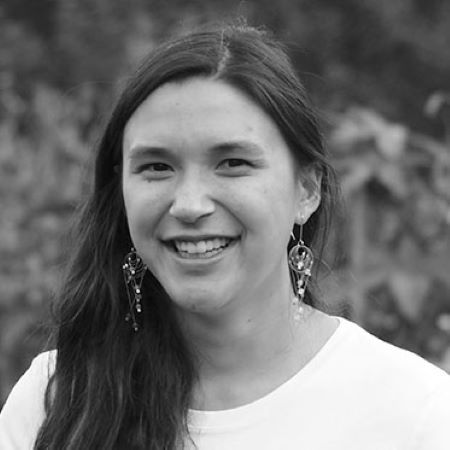
DATE: Fri, June 12, 2020 - 3:30 pm
LOCATION: Please register to receive the Zoom link
DETAILS
Please register for this event here.
Abstract:
Average-case analysis computes the complexity of an algorithm averaged over all possible inputs. Compared to worst-case analysis, it is more representative of the typical behavior of an algorithm, but remains largely unexplored in optimization. One difficulty is that the analysis can depend on the probability distribution of the inputs to the model. However, we show that almost all instances of high-dimensional data are indistinguishable to first-order algorithms. Particularly for a class of large-scale problems, which includes random least squares and one-hidden neural networks with random weights, the halting time is independent of the probability distribution. With this barrier for average-case analysis removed, we provide the first explicit average-case convergence rates showing a tighter complexity not captured by traditional worst-case analysis. Finally, numerical simulations suggest this universality property holds in greater generality. Joint work with Elliot Paquette, Fabian Pedregosa, and Bart van Merriënboer
Bio:
Courtney Paquette is a research scientist at Google Research, Brain. Paquette’s research broadly focuses on designing and analyzing algorithms for large-scale optimization problems, motivated by applications in data science. She received her PhD from the mathematics department at the University of Washington (2017) and held postdoctoral positions at Lehigh University (2017-2018) and an NSF postdoctoral fellowship at the University of Waterloo (2018-2019). She will be starting an assistant professorship at McGill University in the fall and she will be a CIFAR Canada AI chair with the Quebec AI institute (MILA).
Please register for this event here.