AI & Fundamentals
Learning Generative Models of 3D Shapes: From Implicit Functions to Structured Representations - Richard (Hao) Zhang, Professor, SFU
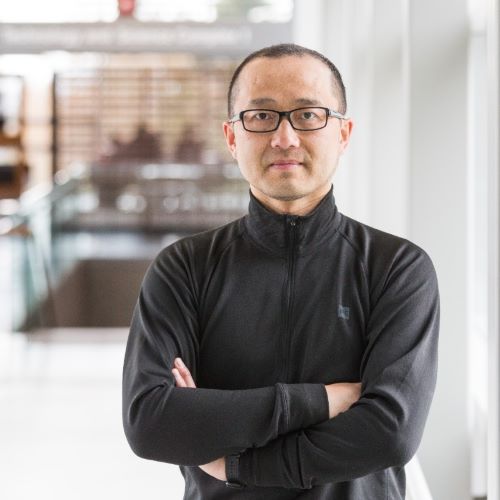
DATE: Mon, February 22, 2021 - 1:00 pm
LOCATION: Please register to receive the Zoom link
DETAILS
Please register for this event here.
Abstract:
Unlike images and video, 3D shapes are not confined to one standard representation. This is one of the challenges we face when developing deep neural networks (DNNs) to learn generative models of 3D shapes or virtual scenes. So far, voxel grids, multi-view images, point clouds, and integrated surface patches have all been considered. In this talk, I show that traditional convolutional neural networks operating on pixels/voxels may not be best suited for the task. I first present IM-Net, our recent work on learning implicit functions, and show the innate ability of implicit models for shape generation and 3D reconstruction. Interestingly, a small tweak of IM-NET, with an added branching layer, would allow the new network, BAE-Net, to perform unsupervised shape segmentation. Furthermore, by explicitly exploiting the interpretability of BAE-Net, we arrive at BSP-Net (BSP for binary space partitioning), a DNN which directly outputs compact, structured meshes, with sharp features. While the IM-BAE-BSP-Net series exemplify the power of "deep shape priors" for part extraction, these works do not learn part relations. In the second part of my talk, I present a generative recursive autoencoder to learn shape structures in the form of attributed trees. Such an approach decouples coarse and fine-grained learning of structured data, which is applicable to both 3D shapes, indoor scenes, and digital documents.
Bio:
Richard (Hao) Zhang is a professor in the School of Computing Science at Simon Fraser University (SFU), Canada, and holds a Distinguished University Professorship (2020-25). He has also been a visitor professor at Stanford University, Shenzhen University, and the Beijing Film Academy. Richard obtained his Ph.D. from the University of Toronto, and MMath and BMath degrees from the University of Waterloo. He directs the GrUVi (Graphics U Vision) lab at SFU. His research is in computer graphics and visual computing with special interests in geometric modeling, shape analysis, 3D vision, geometric deep learning, as well as computational design and fabrication. He has published more than 170 papers on these topics and methods from three of his papers on geometry processing have been adopted by CGAL, the open-source Computational Geometry Algorithms Library. Richard has received several awards including an NSERC Discovery accelerator Supplement in 2014, a Faculty of Applied Sciences Research Excellence Award at SFU in 2014, a National Science Foundation of China (NSFC) Overseas Outstanding Young Researcher Award in 2015, a Google Faculty Research Award in 2019, as well as faculty grants/gifts from Adobe and Autodesk. He and his students have won the CVPR 2020 Best Student Paper Award and Best Paper Awards at CAD/Graphics 2017 and SGP 2008.
Please register for this event here.