AI & Fundamentals
Operationalizing Counterfactual Metrics: Incentives, Ranking, and Information Asymmetry - Serena Wang, PhD Student, UC Berkeley
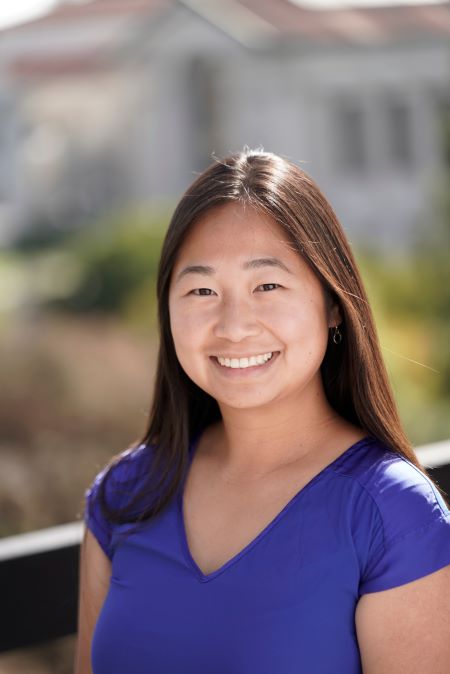
DATE: Thu, November 30, 2023 - 10:30 am
LOCATION: UBC Vancouver Campus, ICCS X836
DETAILS
Abstract:
From the social sciences to machine learning, it has been well documented that metrics to be optimized are not always aligned with social welfare. In healthcare, Dranove et al. (2003) showed that publishing surgery mortality metrics actually harmed the welfare of sicker patients by increasing provider selection behavior. We analyze the incentive misalignments that arise from such average treated outcome metrics, and show that the incentives driving treatment decisions would align with maximizing total patient welfare if the metrics (i) accounted for counterfactual untreated outcomes and (ii) considered total welfare instead of averaging over treated patients. Operationalizing this, we show how counterfactual metrics can be modified to behave reasonably in patient-facing ranking systems while preserving incentive-alignment properties. Extending to realistic settings when the providers observe more about patients than the regulatory agencies do, we bound the decay in performance by the degree of information asymmetry between a principal and agent. In doing so, our model connects principal-agent information asymmetry with unobserved heterogeneity in causal inference.
Bio:
Serena is a final-year PhD student in Computer Science at UC Berkeley. She has also concurrently worked at Google Research at 20% time for the last six years, where she is part of the Discrete Algorithms Group. Serena’s research focuses on understanding and improving the long-term societal impacts of machine learning by rethinking ML algorithms and their surrounding incentives and practices. Serena is particularly interested in gaps between metrics and goals, and how those gaps may be bridged through algorithmic improvements, analysis of multi-agent incentives, and better qualitative understanding of problem formulation. Serena employs tools from machine learning, robust optimization, causal inference, and most recently, microeconomics. Serena is a recipient of the NSF Graduate Research Fellowship and the Apple Scholars in AI/ML PhD fellowship.