AI & Fundamentals
When Should Reinforcement Learning Use Causal Reasoning? - Oliver Schulte, Professor, Simon Fraser University
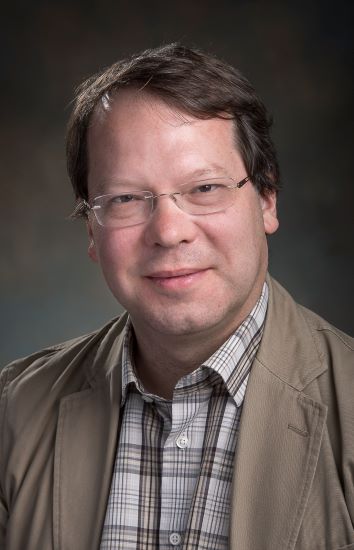
DATE: Mon, November 18, 2024 - 1:00 pm
LOCATION: UBC Vancouver Campus, ICCS X836 / Zoom
DETAILS
Abstract:
Reinforcement learning (RL) and causal modelling naturally complement each other. The goal of causal modelling is to predict the effects of interventions in an environment, while the goal of reinforcement learning is to select interventions that maximize the
rewards the agent receives from the environment. Reinforcement learning includes the two most powerful sources of information for estimating causal relationships: temporal ordering and the ability to act on an environment. This paper examines which reinforcement learning settings we can expect to benefit from causal modelling, and how. In online learning, the agent has the ability to interact directly with their environment, and learn from exploring it. Our main argument is that in online learning, conditional probabilities are causal, and therefore offline RL is the setting where causal learning has the most potential to make a difference. Our paper formalizes this argument. For offline and hybrid offline/online RL, we describe previous and new methods for leveraging a causal model.
Bio
Oliver Schulte is a Professor in the School of Computing Science at Simon Fraser University, Vancouver, Canada. He received his Ph.D. from Carnegie Mellon University in 1997. He has published papers in leading AI and machine learning venues on a variety of topics, including learning causal models and applications of reinforcement learning in sports analytics. While he has won some nice awards, his biggest claim to fame may be a draw against chess world champion Gary Kasparov.