AI & Fundamentals
Diffusion Models: From Foundations to Image, Video and 3D Content Creation - Karsten Kreis, Senior Research Scientists, NVIDIA’s Toronto AI Lab
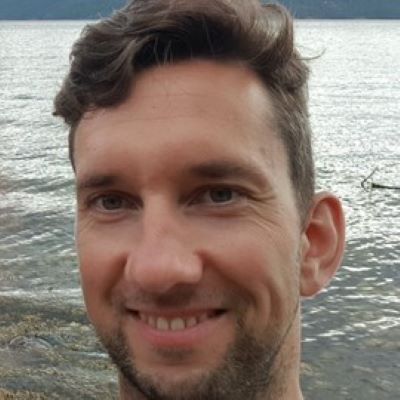
DATE: Tue, July 18, 2023 - 11:00 am
LOCATION: UBC Vancouver Campus, ICCS X836 / Please register to receive Zoom link
DETAILS
Abstract:
Denoising diffusion-based generative models have led to multiple breakthroughs in deep generative learning. In this talk, I will provide an overview over recent works by the NVIDIA Toronto AI Lab on diffusion models and their applications for digital content creation. For the first part of my presentation, I will start with a short introduction of diffusion models and recapitulate their mathematical formulation. Then, I will briefly discuss some of our foundational works on diffusion models, which includes advanced diffusion processes for faster and smoother diffusion and denoising as well as techniques for more efficient model sampling. In the second part, I will discuss works that use diffusion models for image, video and 3D content creation. This includes large text-to-image models as well as recent work on high-resolution video synthesis with latent diffusion models. I will also summarize some of our efforts on 3D generative modeling. This includes object-centric 3D synthesis by training diffusion models on geometric shape datasets or leveraging large-scale text-to-image diffusion models as priors for shape distillation, as well as full scene-level generation with hierarchical latent diffusion models.
Bio:
Karsten Kreis is a senior research scientist at NVIDIA’s Toronto AI Lab. Prior to joining NVIDIA, he worked on deep generative modeling at D-Wave Systems and co-founded Variational AI, a startup utilizing generative models for drug discovery. Before switching to deep learning, Karsten did his M.Sc. in quantum information theory at the Max Planck Institute for the Science of Light and his Ph.D. in computational and statistical physics at the Max Planck Institute for Polymer Research. Currently, Karsten's research focuses on developing novel generative learning methods and on applying deep generative models on problems in areas such as computer vision, graphics and digital artistry, as well as in the natural sciences.