AI & Applications
Large-scale federated and privacy-preserving Evaluation & Analysis Platform (LEAP) - Aline Talhouk, Assistant Professor, UBC
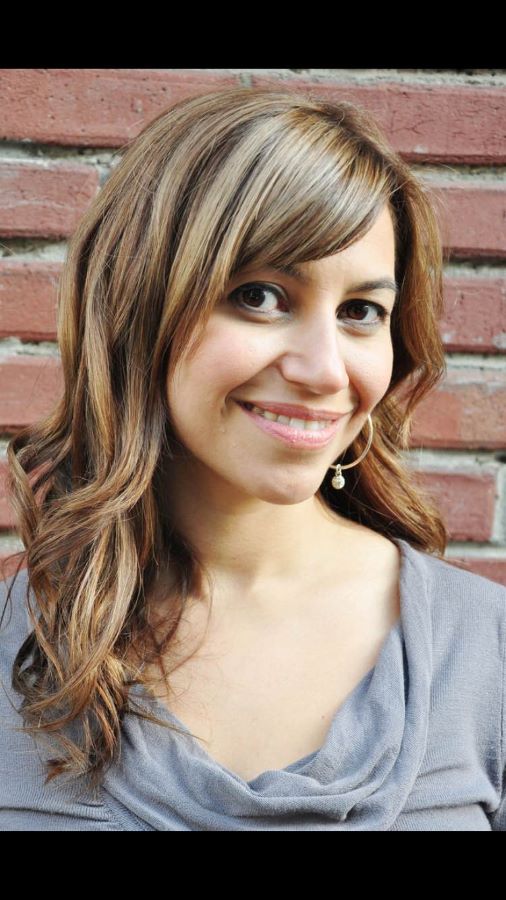
DATE: Fri, January 24, 2020 - 1:00 pm
LOCATION: ANGU 037 - 2053 Main Mall, Vancouver, BC V6T 1Z2
DETAILS
Abstract:
Background: Learning from medical data can enable personalization of patient treatment and improve understanding of disease. Health data are naturally distributed across institutions, but traditionally had to be centralized to allow analysis. Broad and indiscriminate data centralization is not only inefficient, but also at odds with patient privacy, and thus constitutes a barrier to machine learning and analytics of health data.
Objectives: We propose LEAP, a socio-technical solution to analyze distributed medical data, while guaranteeing patient privacy. LEAP combines innovations in computer science, statistics, machine learning, and distributed computing, to implement privacy by design and by default.
Methods: The LEAP prototype we have developed implements Federated Learning (FL), a type of multi-party computation, to help with scalability of machine learning tasks that train over a network and avoid data centralization. We have also implemented Differential Privacy (DP), a procedure that guarantees that a sequence of queries against a database cannot reveal whether a particular record is present in the database. Active development of open source libraries for FL and DP by Google and IBM, signals that these techniques are important to develop and apply in a variety of contexts. However, there is a gap between these general-purpose libraries and what is required to support the healthcare use case.
Results We have built a prototype of LEAP that implements FL and DP in a deployable open source system. Unlike prior systems, LEAP has built-in flexibility because it allows sites to solve data integration separately, does not mandate a particular data model, supports DP natively, and allows querying from different programming languages.
Next steps We will test LEAP with different applications, such as digital pathology, genomics, and symptom data. We will also implement approaches to optimize DP privacy budgets, and communicate differential privacy to lay audiences. As we socialize concepts of distributed computing and differential privacy with different ethic boards, IT and governance stakeholders from different institutions, we will begin to formally explore requirement for deploying LEAP across several institutions within Canada and the United States.
Bio:
Dr. Talhouk is an assistant professor in the department of Obstetrics & Gynecology in the Faculty of Medicine at the University of British Columbia. She is also the director of data science and informatics at OVCARE, BC’s ovarian and gynecological cancer research program. She completed her PhD in Statistics at the University of British Columbia in 2013 with a focus on computational statistics and machine learning. Since then, she has been working on developing and implementing predictive models to improve patient care in women’s health and oncology. Her research focus also includes the ethics of data sharing and privacy in the era of digital health and AI modeling.