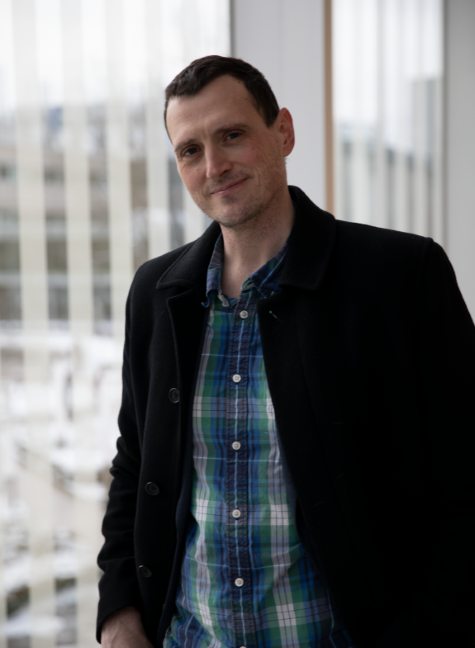
Lithium is a finite resource, but our need for it has never been greater. Lithium batteries are used in everything from your smart phones to electric vehicles. Unfortunately, the very high lithium purity required for batteries is challenging to achieve through current mining and refinement processes.
Current techniques can use up to 500,000 gallons of water just to extract one tonne of lithium, and mining effluent runoff can contaminate our natural environment. Once extraction is complete, the post-mining process normally requires the lithium to be shipped internationally to be refined and is then bought back. The refining process itself involves a lot of trial and error, as the quality of excavated lithium and the amount of impurities is often inconsistent.
The Hein Lab is collaborating with an industry partner, Standard Lithium, to develop a new method that circumvents these challenges. Standard Lithium is a Vancouver-based mining company focusing on extracting lithium from waste and natural brines. They currently extract lithium from the tail brines of a bromine plant in Southern Arkansas. To achieve the very high level of purity required for batteries, though, these extracts must be refined to remove other contaminants. The problem is that many of these materials have very similar solubilities to lithium, making it difficult to separate them from each other – hence why the refining process is normally so taxing. The Hein Lab specializes in using crystallization to separate materials with very similar solubilities, and they are now applying that knowledge to the lithium problem.
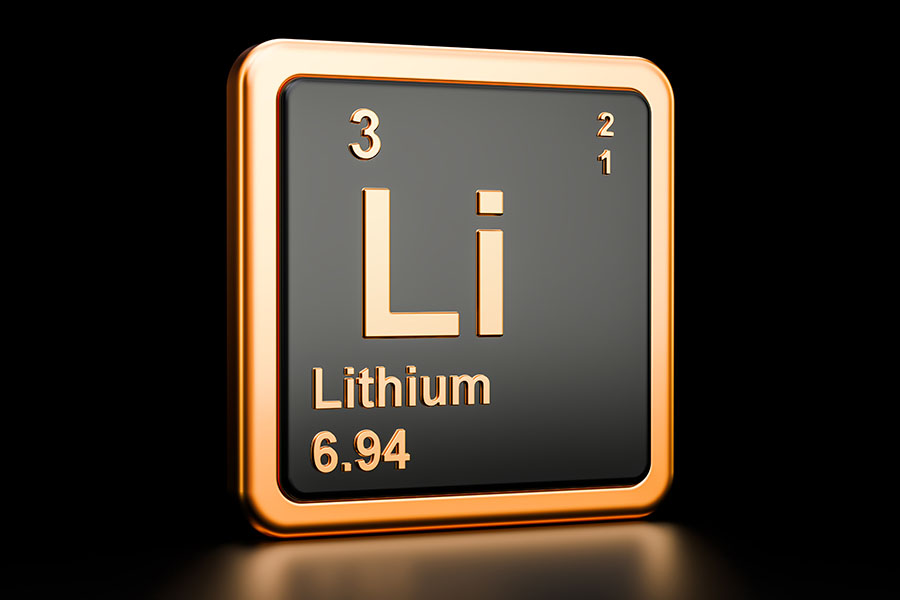
Normally crystallization involves taking all of your dissolved material and heating it or cooling it until solids crystallize. Rapid crystallization would be time efficient, but the faster you cause crystallization, the more likely it is that high energy particles and impurities will hang onto the crystals, preventing you from getting the clarity that you’re looking for. What you take from the ground is never going to be the exact same mixture of materials; therefore, it becomes very difficult to find the magic speed to heat or cool your samples and consistently produce high-quality crystals. In order to get lithium to the point where it is battery-grade, your process has to consistently produce very high purity crystals, which is why the standard procedure involves multiple rounds of measuring and reprocessing. Obviously, this is not ideal. Dr. Hein’s system, instead, evaluates the composition of the incoming material and uses an in-situ camera to monitor the dynamic crystallization process. They can take pictures of the crystals as they’re forming and automatically adjust conditions accordingly, in real time. This smart automation gives control back to the processors and guarantees over 99% lithium purity every time.
What does this mean? Well, essentially, Dr. Jason Hein and his team are taking garbage and making batteries from it; a process that will become even more sustainable down the road if they reach their eventual goal of using atmospheric CO2 for the crystallization process instead of the sodium carbonate that they are currently using. Their process can also be brought online three times faster than traditional extraction schemes, and it can be added to existing oil and gas plants. It is even possible that this technology will enable the first lithium plant in the past 60 years to open in North America, given favourable techno-economics.
This isn’t the only sustainable project the Hein Lab is working on, though; they recently co-authored a paper on a self-driving laboratory to accelerate the discovery and optimization of clean energy materials. This collaboration between Dr. Curtis Berlinguette (UBC), Dr. Jason Hein, and Dr. Alán Aspuru-Guzik (University of Toronto) is known as Project Ada and utilizes both machine learning and automation to speed up materials discovery while reducing experimental bias.
Through reinforcement learning, the team has set up a robotics system that can make a material, analyze it, and learn from it: all without a human present. It can be complicated to design the experiment, as the robot doesn’t have the same common sense that we do, but after the set up is complete the learning algorithm dictates how each experimental round will differ from the last and continues with the next sample. They are currently using this custom system to design and study thin-films for clean energy applications, but this “self-driving lab” concept has the potential to be applied very broadly.
You can learn more about Project Ada here.
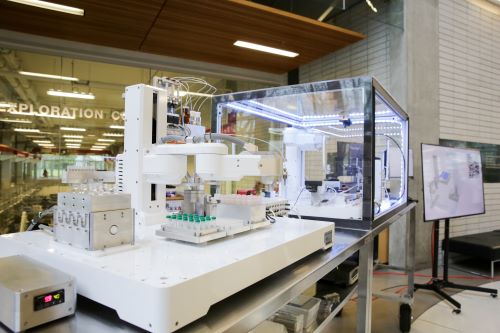
Hein’s team is large and highly interdisciplinary, containing a variety of organic and analytical chemists, mechanical and software engineers, and computer scientists. Dr Hein’s reason for this: they can internally touch on a lot of different areas and it allows them to do more of the project without external collaborators, thereby speeding things up and allowing them to do more. I, for one, am excited to see what this close-knit and diverse team will get up to next.
Article written by Arynn Keane
Content, photos, and editing contributed by the Hein Lab