AI & Fundamentals
New Advances in Safe and Efficient Large Language Models - Hongyang Zhang, Assistant Professor, University of Waterloo
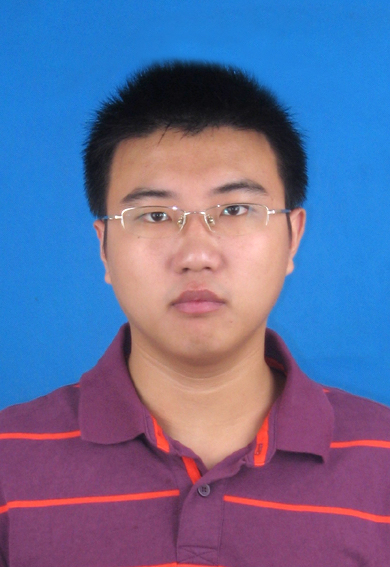
DATE: Wed, February 21, 2024 - 11:00 am
LOCATION: UBC Vancouver Campus, ICCS X836 / Please register to receive Zoom link
DETAILS
Abstract:
Recent strides in large language models (LLMs) have underscored the critical importance of addressing both AI safety and decoding efficiency. In the first segment of this talk, we delve into the integration of self-evaluation and rewind mechanisms within unaligned LLMs, presenting the Rewindable Auto-regressive INference (RAIN) framework. RAIN empowers pre-trained LLMs to autonomously assess their own outputs, leveraging these evaluations to iteratively refine response generation through self-boosting. Notably, this innovative approach enhances AI safety without necessitating additional alignment data, training, gradient computations, or parameter updates.
Transitioning to the second part of the talk, we delve into strategies aimed at accelerating LLM decoding. Here, we introduce the EAGLE framework, a draft-verification technology founded on feature extrapolation. EAGLE achieves an impressive 3x speedup compared to vanilla LLM decoding while provably maintaining the text distribution. By outlining these advancements, this talk provides valuable insights into the ongoing efforts to enhance both the safety and efficiency of large language models.
Bio:
Hongyang Zhang is a tenure-track assistant professor at University of Waterloo and Vector Institute for AI. He received his PhD in 2019 from the Machine Learning Department at Carnegie Mellon University and completed a Postdoc at Toyota Technological Institute at Chicago. He is the winner of NeurIPS 2018 Adversarial Vision Challenge, CVPR 2021 Security AI Challenger, Amazon Research Award, WAIC Yunfan Award, etc. He also served as an area chair for NeurIPS, ICLR, AISTATS, AAAI, and an action editor for DMLR.